The increasing carbon footprint of AI is a challenge. Here is what we can do about it
Smart AI solutions have plenty of advantages, but they do not come free of cost for the environment. According to Assistant Professor Raghavendra Selvan, the usefulness of technology and its environmental cost cannot be disentangled. It is possible to adjust the resource consumption of AI methods and make them more climate-friendly, he says.
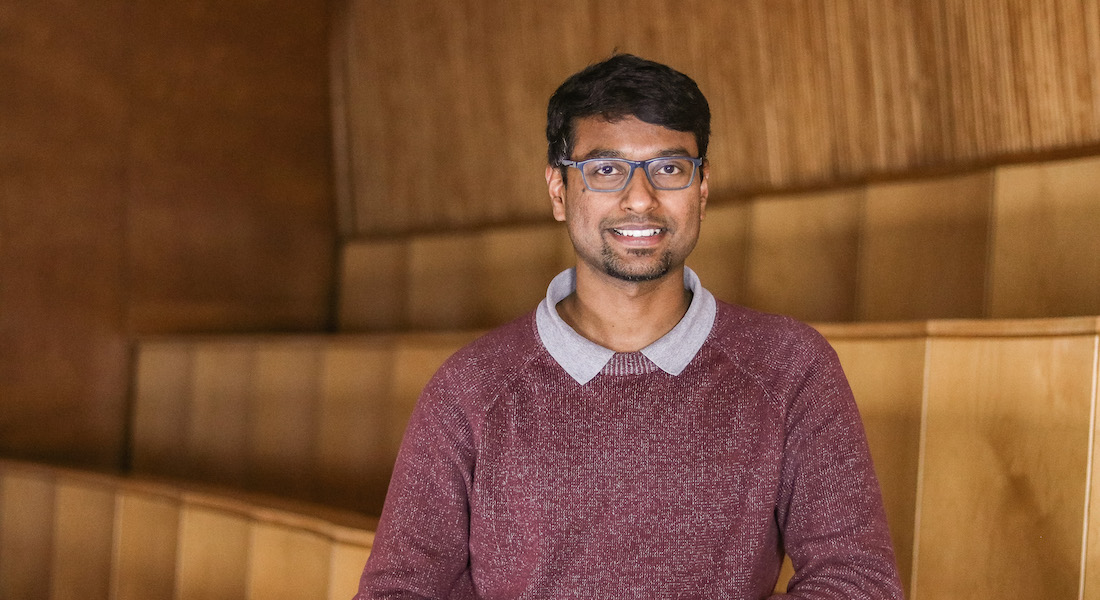
In recent years, we have seen many examples of how AI technology has enabled new discoveries and optimized results and workflows in different areas, from monitoring nature and climate to improving breast cancer risk assessment, often referred to as “smart solutions”.
But there is a cost for being smart. It is estimated that the carbon footprint of the Informations and Communications Technology sector is already at least as much as the aviation sector, and with more and more digitalization and energy-intensive AI solutions, CO2 emissions are expected to grow considerably in the coming years.
According to Tenure-Track Assistant Professor Raghavendra Selvan, we need to become conscious of the environmental cost of deploying AI. He is not advocating for limiting the use of smart solutions, but for finding ways to make them greener while reaping benefits across different applications.
- We often talk about how to use AI methods to advance the United Nations Sustainable Development goals, but we rarely talk about the fact that AI itself has a large underlying resource footprint, including high energy consumption. Even when a technology is used for a green application, it should not prevent us from asking if the technology itself is green or not, says Raghavendra Selvan.
High energy cost = high carbon footprint
In his research, Assistant Professor Raghavendra Selvan investigates ways to lower the carbon footprint of machine learning and create awareness on data costs, which he calls Climate-Aware AI.
- At a microlevel, we can make the algorithms faster and more efficient which would reduce their resource usage. For example, you could look at how to reduce the number of bits used to do the computations, and how to reduce computations that are redundant, says Raghavendra Selvan.
He continues:
- Also, we should continuously assess whether we need all the data that we have stored. The notion of “dark data” refers to the data that is generated but never looked at again. Some estimates suggest that more than half the data stored on data centers is “dark data”. Such data idling on data centers consume energy, and that is redundant.
At a macrolevel, we can look at where and when the computations are done. Choosing non-peak hours, which in Denmark are often powered by wind, for training of AI systems not only reduces the carbon footprint of the training sessions, but it could also reduce the monetary costs, Raghavendra Selvan explains:
- Many computations related to AI system development such as model training are not time critical, which means you can offset these computations by a couple of hours. This can reduce the carbon footprint of these computations as the carbon intensity of electricity can be three times more during peak hours compared to non-peak hours. Further, the monetary cost and the carbon footprint of electricity usually also go hand in hand.
It all starts with awareness
Raghavendra Selvan expects that reporting environmental costs of developing and deploying energy-intensive technology like AI will become a reality in many industries soon. Especially for the data-intensive industries where applying green technology could become an important certification even mandated by laws.
- Some data centres and cloud services are already advertising their products to be greener. We are seeing that custumers who care about their environmental impact choose to use them. But other data sectors have not started doing this, first because the tools for measuring and reporting are not quite mature enough, and second because there is a lack of awareness, Raghavendra Selvan says.
- We already have a lot of awareness of flying less, but when it comes to data, it is not yet common knowledge. I’m hoping that the work my colleagues and I are doing will help make researchers, the industry, and the public conscious about this. It makes it much easier to act on something if you are informed.
Raghavendra Selvan will be speaking about Green ICT with Climate-Aware AI at the Digital Tech Summit 2023 on 8-9 November. You can sign up for free here.
What is the cost of a prompt for ChatGPT?
Here's how he did it:
OpenAI has not published information about how complex the underlying language model of ChatGPT is, plus how many and how powerful computers it is hosted on.
Therefore, Raghavendra Selvan used a similar language model called GPT-J from Huggingface in his study. The GPT3 language model that ChatGPT is based on is 30 times larger than GPT-J.
To measure energy and CO2 emissions, he used the carbontracker tool, which he helped develop.
The hardware he used was a Desktop GPU workstation with Intel i7 processor and 32 GB memory and a Nvidia RTX3090 GPU with 24 GB memory.
The predictions were performed at half precision model (16 bit float instead of 32 bit float) to fit into GPU memory.
Cost of GPT-J per prompt
Raghavendra Selvan made 100 prompts and averaged the energy cost over them. It took about 20 minutes to create 10 prompts without using parallel batch processing. One could also process 10 prompts in parallel (batch processing) 10 times and generate 100 prompts in 20 minutes. For each prompt, the model produced text with an average length of 230 words. Each prompt without parallel processing cost E=0.01292 kWh.
Extrapolation to GPT-3
If the E above is to be scaled to GPT-3, there are two factors to consider:
- Low precision: The overhead of running full precision models compared to half precision is approximately one and a half times greater - so a factor of x1.5
- Difference in the number of parameters: Even if we go by a conservative estimate, the larger model may require 10 additional GPUs. We don't know what hardware ChatGPT is using, but some kind of parallel configuration of multiple GPUs could be more powerful.
The energy cost per request from ChatGpt is therefore E*1.5*10 = 0.1938 kWh or approximately 193.8 Wh. That's the equivalent of charging your mobile phone up to 40 times.
Efficiency could mean lower CO2 emissions
Raghavendra Selvan's study found that on average, one prompt consumes 0.19 kWh. However, he expects OpenAI to have an efficient data centre and a highly optimised configuration. If OpenAI's hardware and software is better optimised than what has been used in this study, a single prompt to ChatGPT will at best be equivalent to charging your mobile phone once, not necessarily the 40 times.
Contact
Raghavendra Selvan
Tenure-track Assistant Professor
Department of Computer Science, University of Copenhagen
raghav@di.ku.dk
Caroline Wistoft
Communications Consultant
Department of Computer Science, University of Copenhagen
cawi@di.ku.dk