Computer scientists show the way: AI models need not be SO power hungry
The development of AI models is an overlooked climate culprit. Computer scientists at the University of Copenhagen have created a recipe book for designing AI models that use much less energy without compromising performance. They argue that a model’s energy consumption and carbon footprint should be a fixed criterion when designing and training AI models.
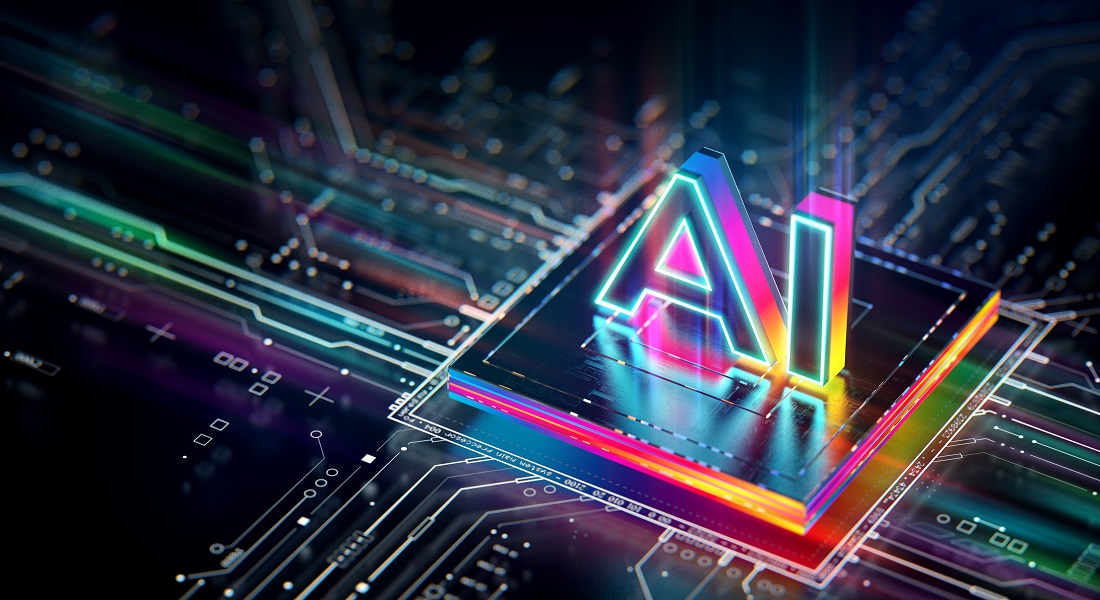
The fact that colossal amounts of energy are needed to Google away, talk to Siri, ask ChatGPT to get something done, or use AI in any sense, has gradually become common knowledge. One study estimates that by 2027, AI servers will consume as much energy as Argentina or Sweden. Indeed, a single ChatGPT prompt is estimated to consume, on average, as much energy as forty mobile phone charges. But the research community and the industry have yet to make the development of AI models that are energy efficient and thus more climate friendly the focus, computer science researchers at the University of Copenhagen point out.
"Today, developers are narrowly focused on building AI models that are effective in terms of the accuracy of their results. It's like saying that a car is effective because it gets you to your destination quickly, without considering the amount of fuel it uses. As a result, AI models are often inefficient in terms of energy consumption," says Assistant Professor Raghavendra Selvan from the Department of Computer Science, whose research looks in to possibilities for reducing AI’s carbon footprint.
But the new study, of which he and computer science student Pedram Bakhtiarifard are two of the authors, demonstrates that it is easy to curb a great deal of CO2e without compromising the precision of an AI model. Doing so demands keeping climate costs in mind from the design and training phases of AI models.
"If you put together a model that is energy efficient from the get-go, you reduce the carbon footprint in each phase of the model's 'life cycle'. This applies both to the model’s training, which is a particularly energy-intensive process that often takes weeks or months, as well as to its application," says Selvan.
Recipe book for the AI industry
In their study, the researchers calculated how much energy it takes to train more than 400,000 convolutional neural network type AI models – this was done without actually training all these models. Among other things, convolutional neural networks are used to analyse medical imagery, for language translation and for object and face recognition – a function you might know from the camera app on your smartphone.
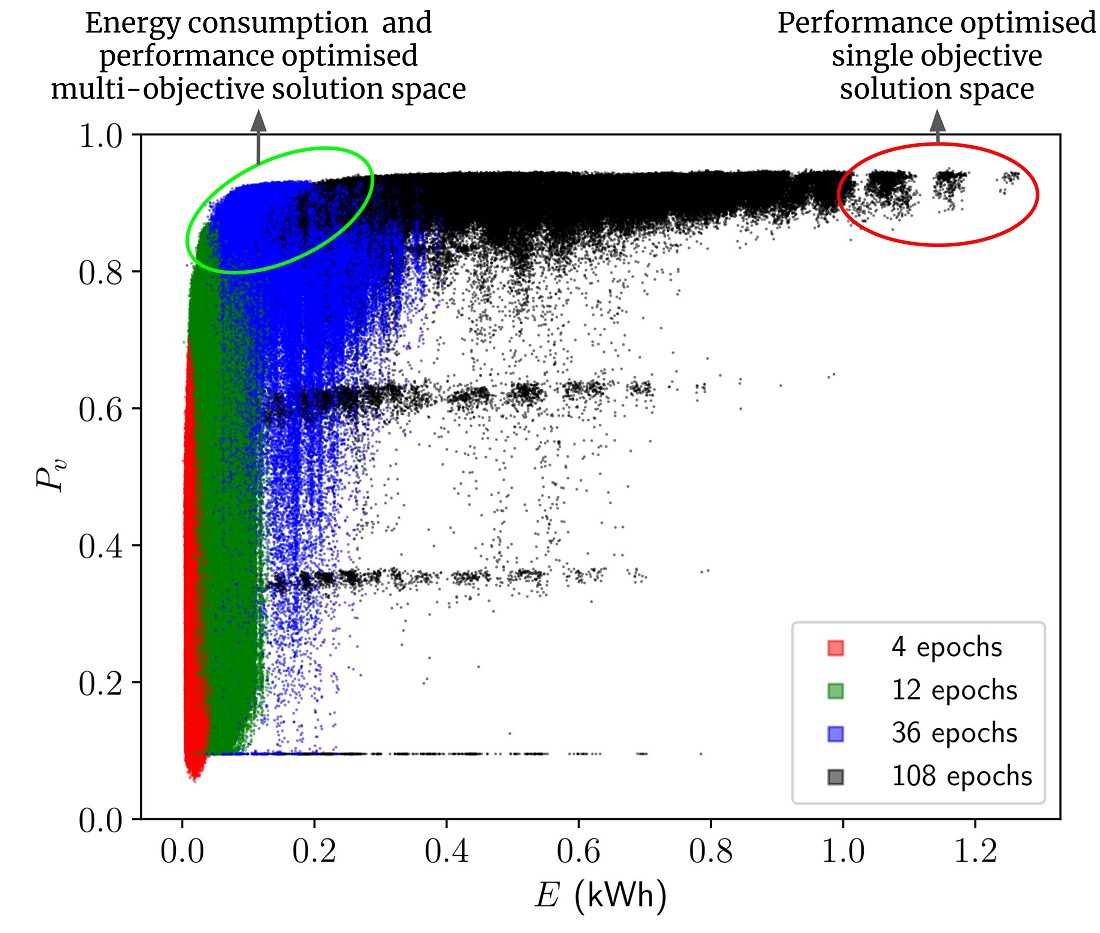
Each dot in this figure is a convolutional neural network model with the energy consumption on horizontal axis and performance on vertical axis. Conventionally, models are selected only based on their performance - without taking their energy consumption into account - resulting in models in the red ellipse. This work enables practitioners to select models from the green ellipse which give good trade-off between effectiveness and efficiency.
Based on the calculations, the researchers present a benchmark collection of AI models that use less energy to solve a given task, but which perform at approximately the same level. The study shows that by opting for other types of models or by adjusting models, 70-80% energy savings can be achieved during the training and deployment phase, with only a 1% or less decrease in performance. And according to the researchers, this is a conservative estimate.
"Consider our results as a recipe book for the AI professionals. The recipes don’t just describe the performance of different algorithms, but how energy efficient they are. And that by swapping one ingredient with another in the design of a model, one can often achieve the same result. So now, the practitioners can choose the model they want based on both performance and energy consumption, and without needing to train each model first," says Pedram Bakhtiarifard, who continues:
"Oftentimes, many models are trained before finding the one that is suspected of being the most suitable for solving a particular task. This makes the development of AI extremely energy-intensive. Therefore, it would be more climate-friendly to choose the right model from the outset, while choosing one that does not consume too much power during the training phase."
The researchers stress that in some fields, like self-driving cars or certain areas of medicine, model precision can be critical for safety. Here, it is important not to compromise on performance. However, this shouldn’t be a deterrence to striving for high energy efficiency in other domains.
"AI has amazing potential. But if we are to ensure sustainable and responsible AI development, we need a more holistic approach that not only has model performance in mind, but also climate impact. Here, we show that it is possible to find a better trade-off. When AI models are developed for different tasks, energy efficiency ought to be a fixed criterion – just as it is standard in many other industries," concludes Raghavendra Selvan.
The “recipe book” put together in this work is available as an open-source dataset for other researchers to experiment with. The information about all these 423,000 architectures is published on Github which AI practitioners can access using simple Python scripts.
Contact
Raghavendra Selvan
Assistant Professor
Department of Computer Science
University of Copenhagen
raghav@di.ku.dk
+45 31 87 30 52
Pedram Bakhtiarifard
Master’s student
Department of Computer Science
University of Copenhagen
pba@di.ku.dk
+45 60 21 99 10
Maria Hornbek
Journalist
Faculty of Science
University of Copenhagen
maho@science.ku.dk
+45 22 95 42 83